We've reached peak "stuff."
Shoppers have too many choices, and it's causing choice overload — a phenomenon that occurs when people have too many options 🤯
Left unchecked, choice overload could kill your conversions. More than half of consumers have stopped purchasing products from a brand's online store because of too many choices.
Consumers are basically sending out an SOS. They're telling you: "We don't know what to buy. Help us!"
Fifty-two percent of shoppers expect personalized offers. Recommendations are the best solution to serve up the right products to the right customer at the right time.
Enter product recommenders, aka product recommendation engines.
These tools suggest relevant products to customers based on previous purchases or browsing history. Recommenders use machine learning and artificial intelligence to make personalized suggestions.
A carefully selected recommendation can convert browsers into buyers. AND even increase your average order value.
How Do AI Product Recommenders Work?
Product recommenders analyze user data to learn what items appeal to a specific customer.
An advanced product recommendation engine can create personalized product suggestions based on real-time customer behavior and integrate with various ecommerce platforms.
They collect first-party data (both from the current customer and their entire customer pool). This data includes what the customer's likes, how they use the website, and what similar customers like. Then, they calculate what will appeal most to each customer in the future.
Recommendation engines typically achieve results using one or both types of data filtering. These include content-based and collaborative filtering.
Collaborative filtering combines data from similar users
Collaborative filtering systems analyze data about customers’ individual preferences and pair that info with data from users with similar tastes. Think “customers who bought this item also bought…” recommendations.
Spotify’s popular Discover Weekly playlist uses collaborative filtering systems.
The platform collects data on what a user listens to, the playlists they create, and how often they stream the tracks they enjoy. Then, it compares the user’s habits with data from over 500 million other users to find similarities. Based on these patterns, Spotify creates a curated mixtape of 30 songs it thinks you’ll love.
And judging by the numbers, most listeners love their curated playlists. As of 2020, Spotify users had spent over 2.3 billion hours streaming their Discover Weekly playlists. A testament to how powerful collaborative filtering can be for enhancing the customer experience.
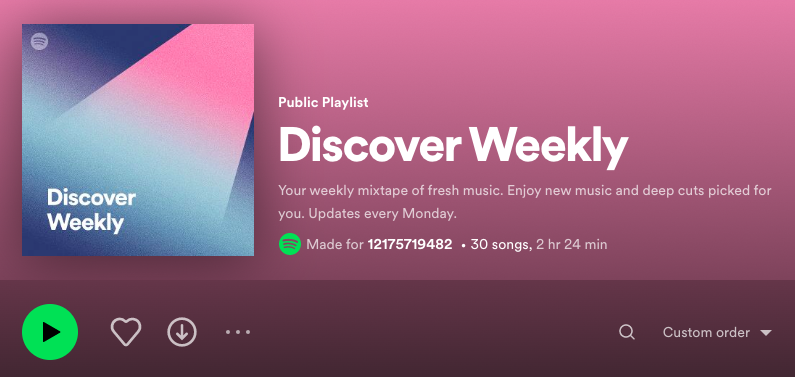
Improves product discovery
Collaborative filtering helps users discover new items they might not have otherwise found. By analyzing a customer's preferences and purchase history, collaborative filtering can suggest products that align with their tastes. Just think of how many times you’ve discovered a new song or artist on Spotify. That’s all thanks to the power of collaborative filtering.
This makes collaborative filtering an excellent tool to use for upselling and cross-selling. You can help customers discover new products or interests. Customers don’t even need to actively search. You can recommend items popular among users with similar tastes at any point in the customer experience.
Can be set up without any previous customer data
Collaborative filtering can suggest items to shoppers even without any data on their purchase history. This capability can enhance the shopping experience and lead to increased customer loyalty over time.
For example, you can show new visitors popular or trending products on your homepage or product pages through recommendation widgets. A widget that says, “Customers who bought this item also bought…” shows products that are often bought together.
Content-based Filtering Uses Previous Interactions With Items
Content-based filtering generates “If you liked this, you might also like that” recommendations. These systems use machine learning algorithms to analyze the content of items and make personalized suggestions.
This system provides personalized recommendations to users based on what they’ve shown interest in. It looks at the item’s details to compare against other items on your site. It then suggests other products based on similarities in the copy or code.
For example, Manssion, a designer of premium men’s jewelry, shows customers a “You may also like” suggestion on the cart page. This includes related products based on items in their shopping carts. Using this widget as part of their product personalization strategy has helped boost Manssion’s AOV by 18.65%
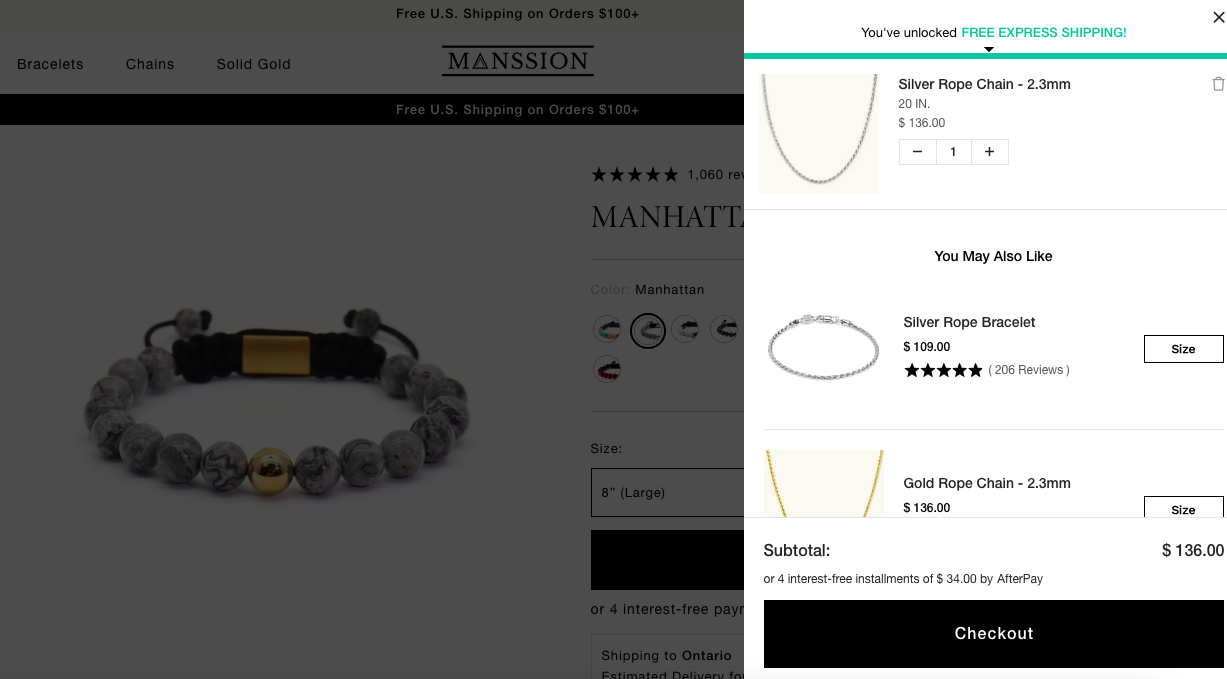
Creates greater transparency
Around three-quarters of customers want companies to be clear about how they use their information. And content-based filtering is more transparent than other recommendation approaches.
Collaborative filtering relies on personal data or browsing history. But content-based filtering doesn’t require this. Instead, it analyzes the content of items directly, such as the products in your shopping cart.
This transparency makes content-based filtering easier to explain to users. Greater transparency can significantly improve customer satisfaction by making users feel more comfortable with the recommendations. If you previously searched for silver chains, you won’t be surprised to see recommendations for silver bracelets.
You don't need much data
When making suggestions to new users, you have limited data for effective product suggestions. But content-based filtering doesn’t need data from other users to create recommendations.
Unlike collaborative filtering, the recommendation engine still provides relevant recommendations even with limited user data.
Hybrid recommendation systems using machine learning algorithms increase the accuracy of recommendations
Hybrid recommendation systems use both content-based and collaborative filtering. This approach can make a significant impact on a business's ecommerce strategy by providing more accurate and diverse recommendations. The result is even more accurate personalized product recommendations.
Netflix, for example, uses a hybrid approach for its recommendation system. It’s so accurate that the platform says personalized recommendations from the engine drive 80% of viewer activity.
Many hybrid systems start with collaborative filtering. Say, to identify popular items among users with similar tastes. Then, content-based filtering further refines those recommendations based on product features.
Another option is to start with content-based filtering to identify items similar to those the user has interacted with. And then collaborative filtering recommends items that similar users have enjoyed.
Let’s take a look at how Netflix does it. The platform uses collaborative filtering to analyze data on user behavior and preferences. This includes viewing history, ratings, and searches. Then they identify groups of users with similar interests. Netflix then combines that data with a user’s viewing history and preferences to make recommendations.
Generates more diverse and personalized recommendations
A hybrid recommendation system combines the strengths of collaborative filtering with content-based filtering. But it also overcomes the limitations of each approach. This makes hybrid systems more accurate than single-method systems.
Hybrid systems also recommend a broader range of items than single-method systems. This can lead to higher user engagement as customers are more likely to find products that interest them. For example, a hybrid system that uses collaborative filtering can recommend popular products. While a system that uses content-based filtering can recommend similar or complementary products.
In addition, combing these two methods across your web pages creates more opportunities to upsell and cross-sell. You can recommend popular products that also complement the user’s purchase.
Offers resilience to cold-start problems
A hybrid system is more resilient to cold-start problems. This resilience can improve customer satisfaction by providing relevant recommendations even to new users. That’s because hybrid systems can use one method to make recommendations for new users or items until there is enough data to use another method. A user who has never visited your site will see best sellers. The next time they return, you’ll have more data and can make more specific recommendations.
Join the AI Revolution With Rebuy
The ecommerce landscape is competitive. Businesses that don’t add product recommenders to their ecommerce merchandising strategy risk falling behind. Product recommenders aren’t just a smart move for your ecommerce site.
They’re necessary for staying ahead of the curve to create a more personalized and satisfying user experience.
Dive deeper and learn more about how AI is revolutionizing ecommerce.
And then, when you’re ready to join the AI revolution, use our AI-powered personalization platform for Shopify and Shopify Plus. It serves up different types of product recommendations to your customers and helps you improve customer engagement and increase sales. Additionally, AI-powered recommendation engines can enhance the effectiveness of email marketing campaigns by providing personalized product suggestions.
•••
Try Rebuy free and see why the world’s top brands use Rebuy to accelerate sales growth.
Interested in partnering with Rebuy? Let's do it.
To keep up with the latest trends, updates, and more, follow us on LinkedIn.